This article heavily references CSDN Backpropagation Algorithm (Process and Formula Derivation)
Basic Definitions#
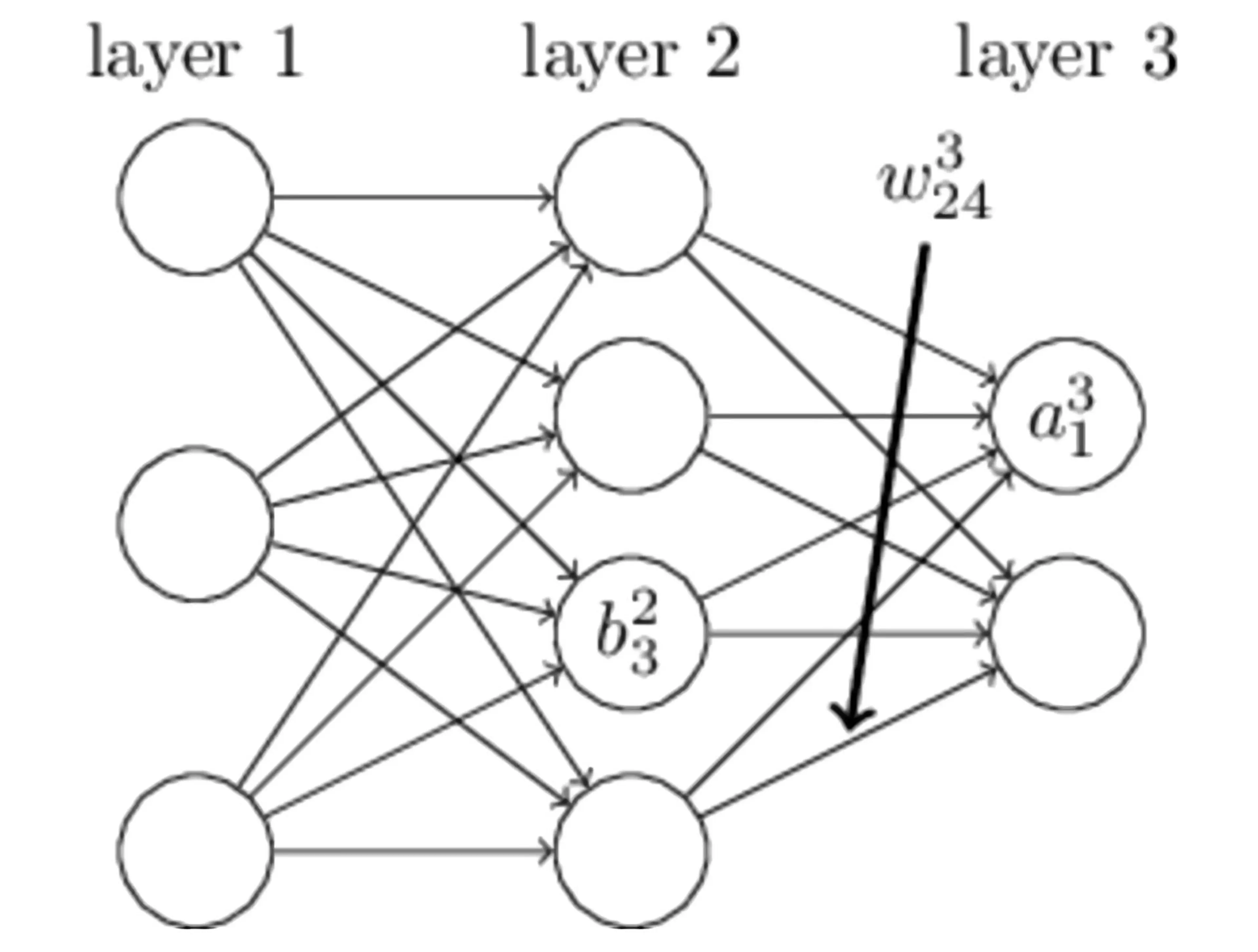
In the simple neural network shown in the above diagram, layer 1
is the input layer, layer 2
is the hidden layer, and layer 3
is the output layer. We use the diagram to explain the meaning of some variable names:
Name | Meaning |
---|
bil | Bias of the i-th neuron in layer l |
wjil | Connection between the i-th neuron in layer l−1 and the j-th neuron in layer l |
zil | Input of the i-th neuron in layer l |
ail | Output of the i-th neuron in layer l |
σ | Activation function |
Based on the above definitions, we can know that:
zjl=∑iwjilail−1+bjl
ajl=σzjl=σ(∑iwjilail−1+bjl)
We define the loss function as the quadratic cost function:
J=2n1∑x∣∣y(x)−aL(x)∣∣2
Where x represents the input sample, y(x) represents the actual classification, aL(x) represents the predicted classification, and L represents the maximum number of layers in the network. When there is only one input sample, the loss function J is denoted as:
J=21∑x∣∣y(x)−aL(x)∣∣2
Finally, we define the error generated in the i-th neuron in the l-th layer as:
δil≡∂zil∂J
Formula Derivation#
The error of the last layer of the neural network with respect to the loss function is:
δiL=∂ziL∂J=∂aiL∂J⋅∂ziL∂aiL=∇J(aiL)σ′(ziL)
δL=∇J(aL)⊙σ′(zL)
The error of the j-th layer of the network with respect to the loss function is:
δjl=∂zjl∂J=∂ajl∂J⋅∂zjl∂ajl=i∑∂zil+1∂J⋅∂ajl∂zil+1⋅∂zjl∂ajl=i∑δil+1⋅∂ajl∂wijl+1ajl+bil+1⋅σ′(zjl)=i∑δil+1⋅wijl+1⋅σ′(zjl)
δl=((wl+1)Tδl+1)⊙σ′(zl)
Therefore, we can calculate the gradient of the weights through the loss function:
∂wjil∂J=∂zjl∂J⋅∂wjil∂zjl=δjl⋅∂wjil∂(wjilail−1+bjl)=δjl⋅ail−1
∂wjil∂J=δjl⋅ail−1
Finally, we can calculate the gradient of the biases through the loss function:
∂bjl∂J=∂zjl∂J⋅∂bjl∂zjl=δjl⋅∂bjl∂wjilail−1+bjl=δjl